Everyone’s Talking AI. The Smart Ones Know: Analytics Comes First — These Stories Show Why. [PART 1]
Trusting your instinct alone is a reckless business gamble. Intuition might strike gold once, but data-driven decision-making doesn’t guess — it knows. - Nnitiwe
In the rush to jump on the latest technological trends, it’s easy to get caught up in the hype. Artificial Intelligence (AI) is the current sensation of the tech world, promising to revolutionize everything from customer service to supply chain management. But here’s a truth that often gets lost in the excitement: AI is not a magic wand.
It’s a powerful tool, yes, but one that requires a solid foundation to be truly effective. That foundation is data analytics.
Why Analytics Before AI?
Imagine trying to build a house without first surveying the land. You might end up with a beautiful structure that collapses because it’s built on unstable ground. Similarly, diving headfirst into AI—whether generative AI or any other kind—without a deep understanding of your data is like building on shaky foundations. AI algorithms are only as good as the data they’re fed and the insights they’re designed to extract. Without a clear grasp of what your data is telling you, you’re essentially flying blind, sabotaging your efforts before they even begin.
Analytics provides the context and clarity needed to make AI work for you, not against you. It helps you identify the right problems to solve, the right data to use, and the right questions to ask. In short, analytics turns raw data into actionable intelligence, setting the stage for AI to shine.
The Limits of Intuition
We’ve all heard stories of business leaders who rely on their gut instincts to make decisions. And while intuition and experience can be valuable assets, they’re not infallible. Human judgment is prone to biases, blind spots, and limitations—especially when dealing with vast amounts of data. What worked in the past might not work in the future, and what seems obvious might not be accurate.
Data-driven decisions, on the other hand, cut through the noise. They reveal patterns and trends that might not be immediately apparent, transforming guesswork into game-changing precision. For instance, a retailer might intuitively stock up on umbrellas when the weather forecast predicts rain. However, data analytics could reveal that customers are more likely to buy raincoats or boots, leading to a more targeted and profitable inventory strategy.
When Analytics Outshines AI
AI is often portrayed as the ultimate solution to every problem, but that’s a misconception. In many cases, simpler analytical methods like data mining or basic statistics can achieve the same results with less complexity and cost.
Applying AI to every challenge is like trying to kill a fly with a gun—overkill and inefficient.
Take customer segmentation as an example. A basic clustering algorithm can group customers based on their purchasing behavior, providing valuable insights for targeted marketing. While Generative AI could certainly be used for this task, it’s often unnecessary. Data mining and analytics can perfectly handle such issues, saving time, resources, and headaches.
Moreover, a strong background in analytics enhances your ability to use AI effectively. It helps you frame the right hypotheses, select the appropriate models, and interpret the results accurately. Advanced AI algorithms hold the power to uncover and solve complex patterns, but without analytics, you risk falling into the trap of overhyped expectations.
The Hidden Goldmine in Your Data
Everyday Data, Surprising Insights…
Data is everywhere—transaction logs, performance metrics, even bullet holes on a plane. When analyzed properly, what seems mundane can unlock game-changing insights.
The following real-world stories show how organizations overcame flawed intuition with data-driven strategies, solving critical problems and transforming their fields. These examples highlight the power of analytics to uncover what intuition misses.
1. Capital One’s Credit Risk Revolution
In the mid-1990s, banks were bleeding money on credit card defaults, stuck in a rigid mindset. The initial intuition was that only “prime” customers—those with excellent credit—were considered safe bets, while others carried too much risk. This led to either rejecting potentially profitable customers or approving too many high-risk ones, costing millions. The problem was clear: traditional credit evaluations couldn’t accurately distinguish between risky and reliable borrowers, leaving banks like Capital One struggling to grow profitably.
In 1994, in McLean, Virginia, under CEO Richard Fairbank, Capital One took a radical approach. The strategy involved mining vast datasets from credit bureaus and internal transaction histories, using regression models and early machine learning to identify subtle patterns in customer behavior. They targeted “subprime” customers who, despite non-traditional profiles, showed predictable repayment habits. The outcome was stunning: default rates plummeted, and Capital One expanded credit access to millions, fueling its rise as a banking giant. This data-driven pivot set the standard for risk-based pricing, proving intuition alone couldn’t match analytics’ precision. Read more.
2. WWII Aircraft Survivability Analysis
During World War II, U.S. military engineers faced a deadly puzzle. The initial intuition was to armor the parts of returning B-24 bombers riddled with bullet holes, assuming these were the most vulnerable areas. The problem, in 1943 at the U.S. Army Air Forces’ Statistical Research Group in New York, was that this approach wasn’t saving enough planes. Too many bombers were still being shot down.
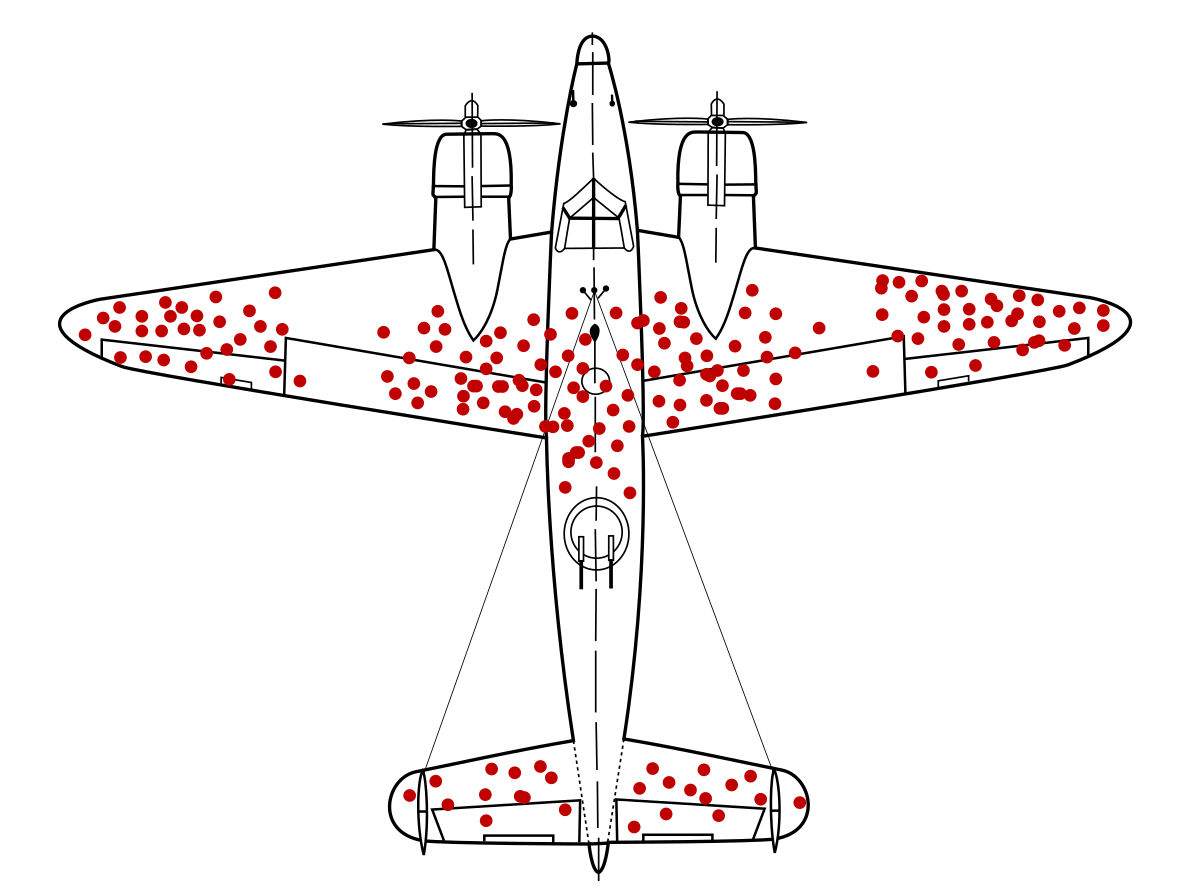
Statistician Abraham Wald flipped the script. The strategy involved analyzing damage patterns on returning planes and recognizing a critical insight: planes hit in less-damaged areas—like engines or cockpits—weren’t making it back. This “survivorship bias” meant the focus should be on protecting those undamaged zones. The outcome was a redesign of armor placement, significantly boosting bomber survivability and saving countless lives. This counterintuitive lesson in missing data remains a cornerstone of analytics education. Read more.
3. British Army Helmet Redesign
Post-World War I, the British Army believed its helmets were top-notch. The initial intuition was that their standard-issue designs, based on engineering assumptions, offered optimal protection. The problem, identified in the 1920s in the UK, was that battlefield injuries suggested otherwise—soldiers were still dying from head wounds at alarming rates.
The strategy involved collecting detailed records of bullet and shrapnel impact sites from battle-damaged helmets. Analysts uncovered patterns showing enemy fire consistently targeted specific zones the helmets didn’t adequately cover. The outcome was a redesigned helmet with improved shape and materials, tailored to these data-driven insights, which slashed head injury rates in subsequent conflicts. This case underscores how analytics can challenge entrenched assumptions, directly impacting lives. Read more.
4. Oakland A’s “Moneyball” Strategy
In 2002, the Oakland Athletics baseball team was cash-strapped and outgunned. The initial intuition among scouts was to chase star players with high batting averages and flashy stats, a strategy the A’s couldn’t afford. The problem was competing against teams like the New York Yankees, who had payrolls triple Oakland’s $41 million budget, in Oakland, California.
General Manager Billy Beane and analyst Paul DePodesta took a data-driven leap. The strategy was to use sabermetrics, focusing on undervalued metrics like on-base percentage (OBP) and slugging percentage, to identify players who could generate runs cheaply. The outcome was a historic 20-game winning streak and a playoff berth, proving small-budget teams could outsmart richer rivals. This revolution forced every MLB team to embrace analytics, reshaping sports forever. Read more.
5. Henry Ford’s Assembly Line Reconfiguration
In the early 1900s, Henry Ford was building cars, but not fast enough. The initial intuition was that giving workers plenty of space and following traditional workflows maximized efficiency. The problem, in 1913 at Ford’s Highland Park plant in Michigan, was that production couldn’t keep up with demand for the Model T, and costs were too high.
Ford’s team turned to data. The strategy involved time-and-motion studies, tracking every step workers took to identify inefficiencies, like excessive walking or poorly placed tools. These insights led to the moving assembly line, with optimized layouts and tool placement. The outcome was revolutionary: production doubled, costs dropped (Model T prices fell from $800 to $350), and Ford set the stage for modern manufacturing. Data trumped tradition, creating an industry standard. Read more.
These stories—from banking to battlefields, sports to factories—show how everyday data, when analyzed with rigor, can overturn flawed assumptions and deliver transformative results. They remind us that the true power of analytics lies in seeing what others miss.
Analytics: Augmenting Human Intelligence
Humans excel at spotting patterns in small, familiar datasets, but our cognitive limits are quickly exposed when grappling with large, chaotic volumes of information. This is where data analytics shines, acting as a force multiplier for human intelligence.
What analytics is: a disciplined process of extracting meaningful insights from data through statistical methods, algorithms, and strategic decision-making. It’s about asking the right questions, choosing the right tools, and knowing when and why to apply them to uncover hidden relationships that drive transformative outcomes.
What analytics isn’t: just plotting bar charts, running exploratory data analysis (EDA), or churning out dashboards for the sake of visualization. While these are tools in the analytics toolkit, true analytics involves understanding the context, defining clear objectives, and interpreting results to inform actionable strategies—not just producing pretty graphs.
This is the power of analytics: it doesn’t replace human intelligence but amplifies it, providing rigorous evidence to validate or challenge assumptions. It transforms raw data into actionable strategies, enabling businesses to operate with precision and foresight.
What’s Next?
In Part 2 of this series, Data for Growth: Proven Ways SMEs Can Use AI & Analytics to Outperform Competitors, we’ll explore how small and medium-sized enterprises can harness analytics to punch above their weight. From optimizing operations to outsmarting larger competitors, we’ll dive into practical strategies and real-world case studies that show how data can be a game-changer for businesses of any size.
Ready to unlock the power of AI and analytics for your business?
Contact me today to assess your AI/analytics readiness and start transforming your data into a competitive edge. Let’s turn insights into impact (👉🏻 send mail to samuel@nnitiwe.io)!
Powerful reminder that AI isn’t a shortcu, it’s a spotlight. Without solid analytics, all it illuminates is noise. The trick is in asking better questions before seeking smarter answers. Analytics isn’t just prep work; it’s the strategic lens that ensures AI delivers impact, not illusion. The WWII survivorship bias case is a timeless masterclass, first heard it in stats class 15 years ago, and it still cuts through noise better than most modern examples. Looking forward to Part 2.
Hello sir… thank you for your interest in my Substack articles… so I visited your post about A.I. being an effective tool for decision making. Fascinating to me as I do not believe logic flow charts are beneficial for our species. I believe we are emotional beings capable of correctly identifying the gait of any individual.
Now I can see the benefits of A.I. in an explorative manner, making better products for civilization or even perhaps to aid entrepreneurs in designing sentient products.
It is your application of A.I. that disturbs me. I have never met a number that could evaluate or even understand what is important in life. As a member of human race I find it insulting to believe that statistics and measurements of profitability is to be trusted more than “Gut” instincts.
A.I. can never, ever be considered a cultural vocation from which to earn money to prospers tribal or even familial goals. It is A.I. vocation is strictly a societal vocation and everyone knows society doesn’t give a shit about anyone as they are in the business of exploiting civilization.
However more disturbing is the fact that this vocation is built on numerical fantasies of being able to diagnose problems and solve them… Why are numbers fantasy? Because I never met a mathematician who could point to nothing… the value they place on the shoulders of 0.
There is a difference between human math and sentient math. Sentient math is able to do what quantum computing can never do… Sentient equations recognize 0 as being everything, not nothing. As a result we are able to account for all variables of relationship between the subject matter that is being explored.
Sentient math is used for exploring possibilities in contrast human math looks to exploit life. But no one needs a calculator in order to survive… instead sentient beings rely on their ability to read gait of all living things. I believe that psychologist call it being a Sigma processing individual.
A.I. will never be able to create passion… but it can produce amazing measurements of probabilities. However I am not subject to probabilities as I am a sentient being. We are not at mercy of some bastard algorithm dictating which course of action to take to survive. No we claim possibilities as our species ruler of voice… Sorry for such a long discourse, however I am after all, passionate.
Question can you give me an example of how A.I. is more qualified to create ideas than a sentient being can use? For humans, I can understand how it would tremendously keep them enslaved as a non thinking aberration of life.